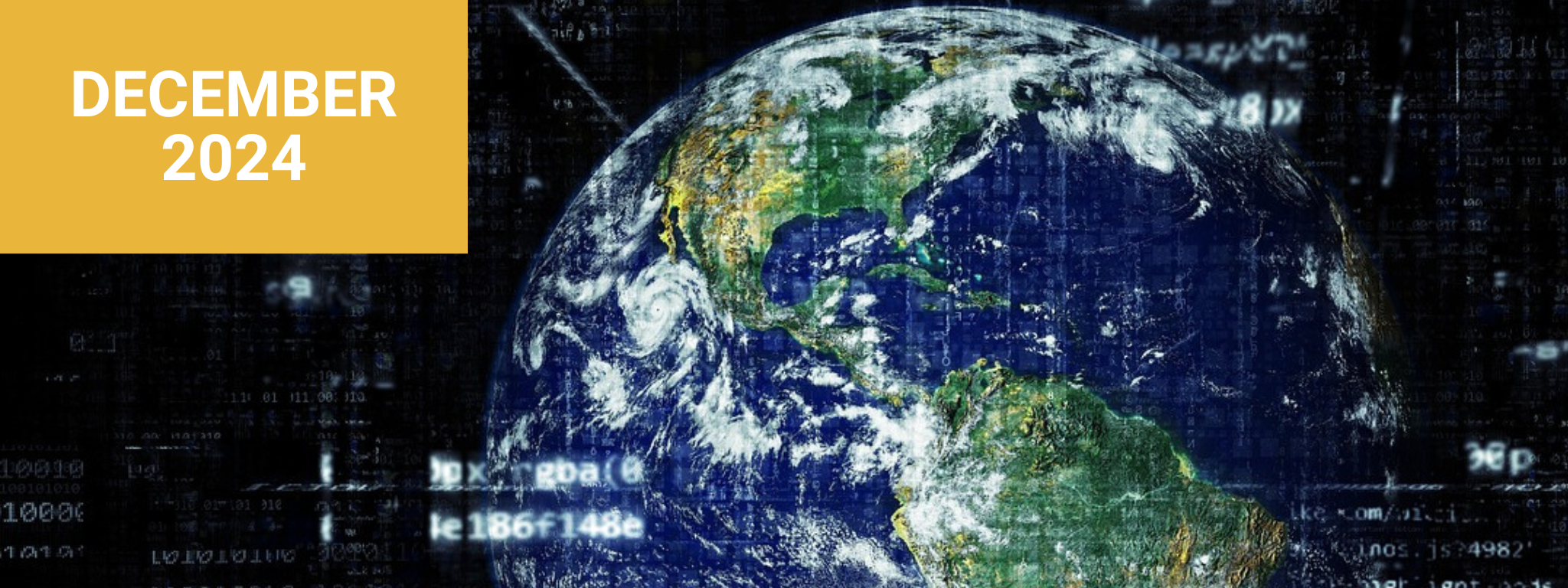
Data Driven Modeling and Predictions of the Earth System
The “Data-Driven Modelling and Predictions of the Earth System” course is designed to equip graduate students with the essential tools and methodologies for understanding and interpreting the complex relationships within Earth system data. As the volume of climate-related data continues to grow, the challenge lies not only in managing this data but in effectively utilizing it to uncover the underlying processes that drive climate dynamics.
This course will offer a deep dive into the mathematical foundations of data-driven modeling, with a particular focus on techniques tailored for analyzing and predicting the behavior of complex dynamical systems.
Bertinoro (FC)
Location
9th – 20th December 2024
Dates
88 h
Total number of hours
20
Number of participants
€ 400,00
Registration Fee
October 24th, 2024
Application deadline
The “Data-Driven Modelling and Predictions of the Earth System” course is designed to equip graduate students with the essential tools and methodologies for understanding and interpreting the complex relationships within Earth system data. As the volume of climate-related data continues to grow, the challenge lies not only in managing this data but in effectively utilizing it to uncover the underlying processes that drive climate dynamics.
This course will offer a deep dive into the mathematical foundations of data-driven modeling, with a particular focus on techniques tailored for analyzing and predicting the behavior of complex dynamical systems. Key topics will include:
1. Hybrid Earth System Models: Students will explore approaches that combine traditional physical models with data-driven techniques to create more accurate and reliable climate predictions.
2. Model Reduction and System Identification: The course will cover methods for simplifying complex models while retaining essential dynamics, as well as techniques for identifying the key components and interactions within the Earth system.
3. Transfer Operators and Uncertainty Quantification: Participants will learn about the estimation of transfer operators, such as the Linear Inverse Models and Koopman operators, which are crucial for understanding the evolution of dynamical systems. The course will also address methods for quantifying and managing uncertainty in predictions.
4. Deep Learning and Control: The course will delve into the application of deep learning in climate science, including techniques for control and optimization of Earth system models.
5. Applications in Climate Science: Practical applications of these methods in climate science and sustainability will be highlighted, demonstrating how data-driven approaches can lead to actionable insights for addressing global climate challenges.
By the end of the program, participants will have a comprehensive understanding of how to leverage data science and machine learning to advance climate research, equipping them to contribute to the next generation of Earth system models and predictions.
This course is designed to address key questions that are essential for understanding the topic:
- How can we effectively integrate data-driven techniques with traditional physical models to enhance the fidelity and accuracy of Earth system predictions?
- What are the best approaches for simplifying complex climate models without losing essential dynamics?
- How can we identify and quantify the underlying processes and interactions within the Earth system using data-driven methods?
- What are the most effective methods for quantifying uncertainty in climate predictions, and how can they be incorporated into data-driven models?
- How can deep learning and control techniques be applied to optimize Earth system models and improve climate predictions?
By the end of the course, participants will have a comprehensive understanding of the following educational objectives:
- Understand the integration of Data-Driven and Physical Models
- Gain proficiency in simplifying complex dynamical systems while preserving critical dynamics and identifying key components and interactions within the Earth system
- Develop Skills in quantifying and representing uncertainty in climate predictions
- Learn the application of deep learning and control methodologies to optimize Earth system models and improve their predictive capabilities.
- Understand the role of transfer operators in analyzing dynamical systems and learn how to estimate and apply these operators to Earth system models.
- Apply the knowledge and skills gained throughout the course to practical problems in climate science and sustainability, developing actionable solutions.
- Engage in interdisciplinary collaboration, working with peers and experts to address complex problems, and effectively communicate their findings.
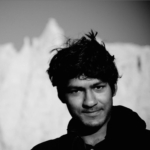
Aneesh Subramanian – Director of the Course
Dr. Aneesh Subramanian earned his Ph.D. in 2012 from Scripps Institution of Oceanography and completed a post-doc there from 2012-2014. He was a Post-Doctoral Research Scientist and Lecturer at the University of Oxford (2014-2017), focusing on advanced weather modeling techniques and their impact on predictability of El Niño, the Madden-Julian Oscillation, tropical cyclones, and atmospheric rivers. He joined the Center for Western Weather and Water Extremes in 2017 as a Project Scientist, enhancing subseasonal prediction and data assimilation for Western US weather. In 2019, he became an Assistant Professor at the University of Colorado Boulder, researching on prediction of our earth system from weather to climate timescales and improving our understanding of climate processes. He has over 90 refereed publications.
His research covers climate processes, global and regional weather and climate prediction, coupled ocean-atmosphere data assimilation and the application of advanced machine learning techniques in prediction and Earth system modeling. He also studies the predictability of global weather systems, and atmospheric rivers on medium-range to sub-seasonal timescales and oceanic influences on prediction in these timescales using both computational models and observational analysis. He is also focused on improved understanding of the physical processes and their representation in computer models for helping improve the predictions.
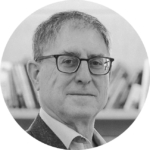
Jeffrey Weiss
Prof. Jeffrey B. Weiss received his Ph.D. in Physics from the University of California, Berkeley, in 1988. He was a postdoctoral fellow and visiting scientist at the National Center for Atmospheric Research in Boulder, CO, before joining the Department of Atmospheric and Oceanic Sciences at the University of Colorado, Boulder in 1993.
Prof. Weiss’s research focuses on applications of dynamical systems and turbulence theory to fundamental problems in the atmosphere, ocean, and climate system, with an emphasis on coherent structures, climate variability, data assimilation, and data driven methods.
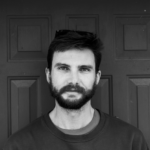
William Chapman
William Chapman is a Project Scientist at the National Center for Atmospheric Research (NCAR) in Boulder, Colorado, working in the Climate & Global Dynamics Group. His research focuses on improving climate and weather predictions by integrating machine learning with traditional climate models. He specializes in subseasonal forecasting, dynamic drivers of climate modes of variability, and error representation within climate models.
His work involves improving the predictability of weather systems, including teleconnection patterns. He aims to enhance forecast skill on medium-range to subseasonal timescales. His research uses a combination of machine learning, computational modeling, and observational analysis to refine our understanding of these processes and improve the representation of physical systems in predictive models.
He earned his Ph.D. in Atmospheric Science from the Scripps Institution of Oceanography. He has held a post-doctoral fellowship at NCAR’s Advanced Studies Program and contributed to the Multiscale Machine Learning (M2lines) project. His work continues to explore the intersection of data-driven methods and traditional climate science, with the goal of enhancing forecasts and deepening our understanding of sources of predictability for extreme weather events.
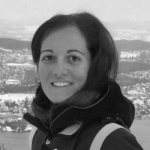
Donata Giglio
Donata Giglio is an Assistant Professor in the Department of Atmospheric and Oceanic Sciences (ATOC) at the University of Colorado Boulder. Her research interests mainly lie in large scale ocean circulation, upper ocean processes and climate dynamics. She also works on improving uncertainty quantification of gridded oceanic fields and in improving ocean data accessibility and visualization via argovis.colorado.edu. She co-chairs the IAPSO best practice study group MapEval4OceanHeat and she is a member of the AMS Committee on Air-Sea Interaction and the UCAR Membership Committee.
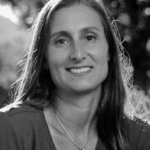
Claire Monteleoni
Claire Monteleoni is a Choose France Chair in AI and a Research Director at INRIA Paris, a Professor in the Department of Computer Science at the University of Colorado Boulder (on leave), and the founding Editor in Chief of Environmental Data Science, a Cambridge University Press journal launched in December 2020. Her research on machine learning for the study of climate change helped launch the interdisciplinary field of Climate Informatics. She co-founded the International Conference on Climate Informatics, which will hold its 14th annual event in 2025. She gave an invited tutorial: Climate Change: Challenges for Machine Learning, at NeurIPS 2014. She currently serves on the U.S. National Science Foundation’s Advisory Committee for Environmental Research and Education, and as Tutorials co-Chair for the International Conference on Machine Learning (ICML) 2024 and 2025.
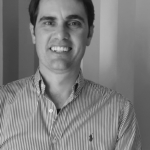
David Lavers
David Lavers is a scientist at the European Centre for Medium-Range Weather Forecasts (ECMWF), working on diagnostics of the ECMWF integrated forecasting system and climate monitoring. His work focuses on the global water cycle, which includes using data from observational campaigns such as Atmospheric River Reconnaissance and the evaluation of precipitation forecasts. He has held previous positions at Scripps Institution of Oceanography, the University of Iowa, the University of Reading, and Princeton University. His PhD on seasonal hydrological prediction was from the University of Birmingham and the Centre for Ecology and Hydrology in the UK.
Location
The course will be at the University Residential Center of Bertinoro (CE.U.B.).
Bertinoro is halfway between the cities of Forlì and Cesena, 6 km from SS9 (Via Emilia). Forlì is the town of reference for transport by train and bus to and from Bertinoro.
Accommodation
The accommodation will be at the University Residential Center of Bertinoro. Please remember that participation in presence is mandatory.
The school is offering limited financial assistance to cover the accommodation costs at the Center of Bertinoro over the entirety of the two-week stay. If you are interested, please check the section below.
For those who will not receive the financial assistance, all the costs will be communicated during the selection process.
The school will provide and offer lunches and dinners for all the participants. Participants are free to organize themselves at their own expense upon notice. The school will not cover any extra costs.
Transport
Nearest airport: Bologna’s airport “Guglielmo Marconi”
Nearest train station: Forlì Station (20 min. far from Bertinoro by car)
On how to get to the Centre, please check this link
Given that most of the participants will arrive in Bologna – especially from abroad – the school will organize a shuttle to bring participants from Bologna to Bertinoro. More details will be given to the selected candidates.
The school offers limited financial assistance based on the applicant’s needs. It will cover accommodation expenses in Bertinoro during the course. If interested, please specify it in the application form in the dedicated section. You can also leave a statement (max 200 words) explaining why you should benefit from this assistance.
The assistance WILL NOT cover travel costs.
Since there is a limited number of places, you are encouraged to apply early to avoid disappointment.
If accepted, you will receive an email confirming your financial assistance by secretariat@fersschool.it
- The maximum age at the time of application is 35 years old.
- The Winter School is mainly geared towards Ph.D. students and postdocs in Mathematics or Climate Science with a strong interest in data science and machine learning.
- Basic programming experience in Python is requested (e.g., numpy, scipy, matplotlib, xarray)